[français]
Low rank matrix denoising for count data with unbiased KL risk estimation
Description
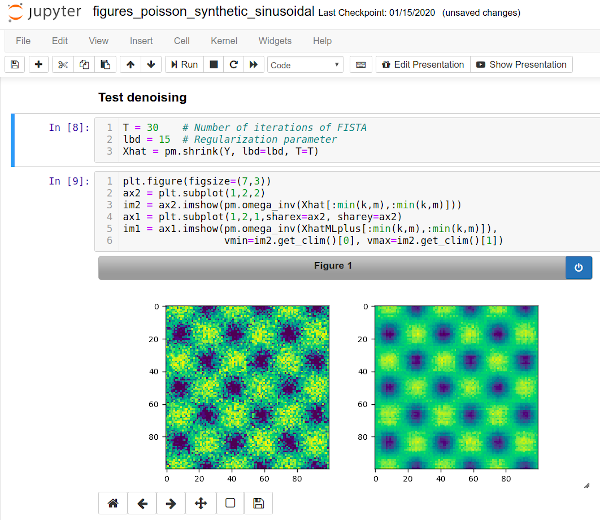
- This software is concerned by the analysis of observations organized in a matrix form whose elements are count data assumed to follow a Poisson or a multinomial distribution. We focus on the estimation of either the intensity matrix (Poisson case) or the compositional matrix (multinomial case) that is assumed to have a low rank structure. We propose to construct an estimator minimizing the regularized negative log-likelihood by a nuclear norm penalty. Our approach easily yields a low-rank matrix-valued estimator with positive entries which belongs to the set of row-stochastic matrices in the multinomial case. Then, our main contribution is to propose a data-driven way to select the regularization parameter in the construction of such estimators by minimizing (approximately) unbiased estimates of the Kullback-Leibler (KL) risk in such models. The evaluation of these quantities is a delicate problem, and we introduce novel methods to obtain accurate numerical approximation of such unbiased estimates. Simulated data are used to validate this way of selecting regularizing parameters for low-rank matrix estimation from count data. Examples from a survey study and metagenomics also illustrate the benefits of our approach for real data analysis.
Associated publications and source codes
Associated publications/reports:-
Jérémie Bigot, Charles Deledalle.
Low rank matrix denoising for count data with unbiased Kullback-Leibler risk estimation
Technical paper (ArXiv)
- Download from:
- Git:
git clone https://bitbucket.org/charles_deledalle/ukla_count_data.git
- Bitbucket: https://bitbucket.org/charles_deledalle/ukla_count_data
- Git:
- Open-source software distributed under CeCILL license
Last modified: Wed Jan 29 08:57:06 UTC 2020