[français]
RABASAR: Ratio-based Multi-temporal SAR Images Denoising
Description
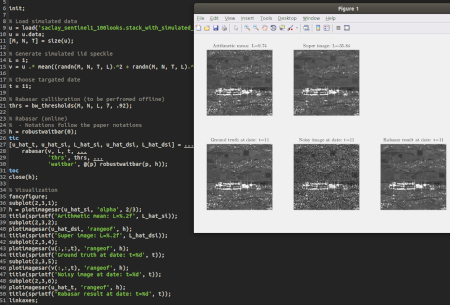
- We propose a fast and efficient multi-temporal despeckling method. The key idea of the proposed approach is the use of the ratio image, provided by the ratio between an image and the temporal mean of the stack. This ratio image is easier to denoise than a single image thanks to its improved stationarity. Besides, temporally stable thin structures are well preserved thanks to the multi-temporal mean. The proposed approach can be divided into three steps: 1) estimation of a "super-image" by temporal averaging and possibly spatial denoising; 2) denoising of the ratio between the noisy image of interest and the "super-image"; 3) computation of the denoised image by re-multiplying the denoised ratio by the "super-image". Because of the improved spatial stationarity of the ratio images, denoising these ratio images with a speckle-reduction method is more effective than denoising images from the original multi-temporal stack. The amount of data that is jointly processed is also reduced compared to other methods through the use of the "super-image" that sums up the temporal stack. The comparison with several state-of-the-art reference methods shows better results numerically (peak signal-noise-ratio, structure similarity index) as well as visually on simulated and real SAR time series. The proposed ratio-based denoising framework successfully extends single-image SAR denoising methods to time series by exploiting the persistence of many geometrical structures.
- Multi-temporal denoising methods take advantage of the increasing availability of SAR image time-series to solve the spatial denoising problems, for the benefit of a better spatial resolution preservation. Most of multi-temporal denoising methods process the whole time series in order to produce a denoised image at a given date. In this paper, we take a different approach by forming a summary of the multi-temporal series (which we call a "super-image", the speckle in that image being strongly reduced), and by using only this "super-image" in addition to the speckle-corrupted image (rather than the whole time series) to obtain a denoised image at any given date. This ratio-based multi-temporal denoising method fully exploits the significant information of the multi-temporal stack through the "super-image". After forming the ratio image between the noisy image and the "super-image", the proposed method takes advantage of the state-of-the-art single-image speckle reduction methods to denoise the ratio image. We consider multi-temporal images acquired on the same orbit (i.e., either all ascending orbits, or all descending orbits), with similar incidence angles, and which have been accurately registered.
- We propose a ratio-based multi-temporal denoising framework. During the restoration of each SAR image, it exploits the temporal information through a super-image. The use of different strategies to compute the super-images has been analyzed. RABASAR can provide better PSNR and MSSIM values when using a spatially denoised super-image. With the increase of the number of images in the time series, the differences of using different super-images decrease. When there are changes in the time series, using a binary weighted arithmetic mean can also provide good results. Based on the processing of time-series corrupted by simulated speckle noise, actual Sentinel-1 stacks and TerraSAR-X stacks, the qualitative and quantitative comparisons with UTA, NLTF, MSAR-BM3D and 2SPPB methods showed the potential of RABASAR to better preserve structures in multi-temporal SAR images while efficiently removing speckle. Besides, the super-image can be easily updated when a new data becomes available so as to process new images on-line.
- See also: MuLoG, NLSAR, NL-InSAR, PPB filter.
Associated publications and source codes
Associated publications/reports:-
Weiying Zhao, Charles-Alban Deledalle, Loïc Denis, Henri Maître, Jean-Marie Nicolas, Florence Tupin.
Ratio-based Multi-temporal SAR Images Denoising: RABASAR
IEEE Transactions on Geoscience and Remote Sensing, vol. 57, no. 6, pp. 3552-3565, 2019 (HAL, IEEE Xplore)
-
Charles-Alban Deledalle, Loïc Denis, Laurent Ferro-Famil, Jean-Marie Nicolas, Florence Tupin.
Multi-temporal speckle reduction of polarimetric SAR images: a ratio-based approach
IGARSS 2019, Yokohama, Japan, July 2019
- Download Python RABASAR 2029 [Mono+Multi-channel] [as of Ref 2.] (recommended):
- Stable version
- Pip:
pip3 install rabasar
- Pypi: https://pypi.org/project/rabasar/
- Bitbucket: https://bitbucket.org/charles_deledalle/rabasar2019-python/src/v0.0.1/
- Pip:
- Development version
- Git:
git clone https://bitbucket.org/charles_deledalle/rabasar2019-python.git
- Bitbucket: https://bitbucket.org/charles_deledalle/rabasar2019-python
- Git:
- Stable version
- Download MATLAB RABASAR 2019 [Mono-channel only] [as of Ref 1.] (unsuported):
- Git:
git clone https://cdeledalle@bitbucket.org/cdeledalle/rabasar.git
- Bitbucket: https://bitbucket.org/cdeledalle/rabasar
- Git:
- Open-source software distributed under CeCILL license
Last modified: Wed Sep 27 04:36:52 UTC 2023